Estimated reading time: 6 minutes
The cost of cybercrime has now outstripped the ability to keep up. Gartner, a multinational security and analytics company in the field of technology, predicted that world spending on cybersecurity will be 16 times lower than damage caused. To address this challenge, organizations are now turning to machine learning and artificial intelligence for cybersecurity, trying to fill in the gaps.
The vast majority of malware targets known vulnerabilities, but botnets often go unnoticed within the victim organizations for around 12 days. The problem in many cases is the scarcity of resources a arrangement.
The attack surface has expanded in recent years with the adoption of IoT devices and the spread of BYOD (Bring Your Own Device) work environments. This, combined with the increasing sophistication of the attacks and increasing the security skills gap, has overwhelmed many teams.
In response, service providers MSSP are increasingly implementing a machine learning system in their cybersecurity solutions. This is certainly an interesting field and many security chiefs are looking for a permanent solution in the fight against cybercriminals. But the question is whether the machine learning offered by artificial intelligence can add new value to the realm of security.
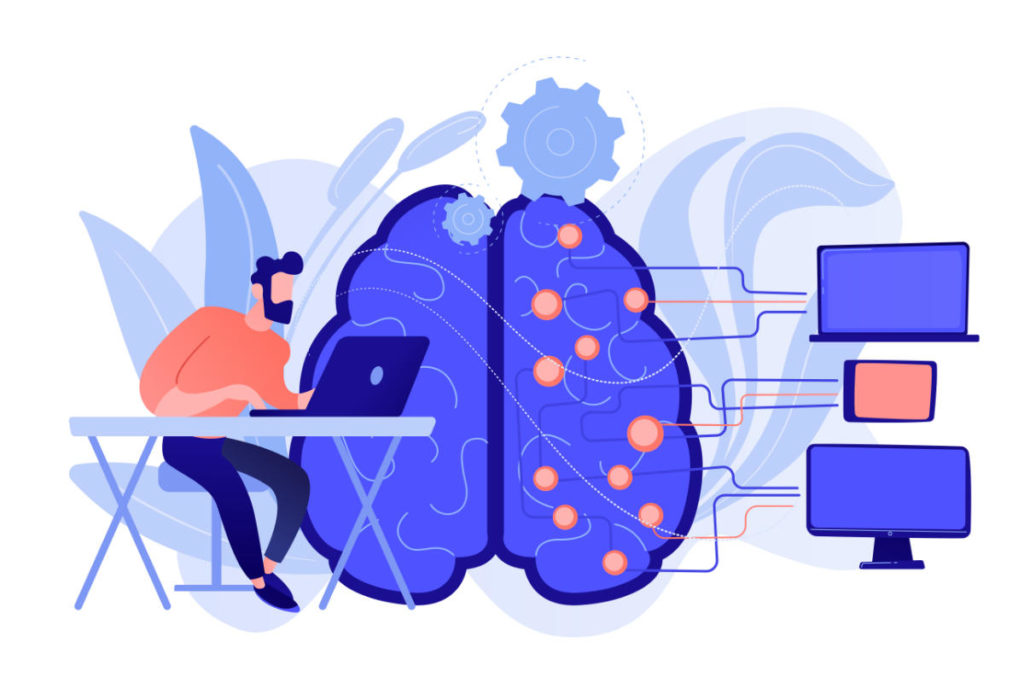
Threat detection and prevention
Most companies are operating with a standard security kit. Their rooms are filled with devices that claim they can detect and prevent the latest threats through signature-based detection, predefined policies or custom configurations. Sensors in this category include firewall , DLP ( Data Loss Prevention ), IPS ( Intrusion Prevention System ) and WCF ( Web Content Filter ) . An alarming consideration, as these sensors continue to be adopted in large numbers, many of them may not be configured correctly .
Furthermore, many of these devices operate in complete isolation , unable to share or correlate information or respond to threats with any kind of coordinated strategy. Consequently, controlling these devices also requires an extra layer of sensors , along with additional members of the security team. Of course, more sensors and more data require more people and given the current security skills shortage, this strategy is unsustainable in the long run.
Through a complete system such as a SOC , however, this does not happen. The collection, correlation and analysis take place in a coordinated way in a SOAR (Security Orchestration, Automation and Response) designed to be a unique workflow. Part of these tasks is done by artificial intelligence and machine learning mess and in the service of cybersecurity . The aim is to analyze user behavior and identify anomalies, as well as learn from past analyzes.
Machine learning for cybersecurity
Machine Learning (ML) is a subset of AI (Artificial Intelligence). AI and ML can enhance our human capabilities by allowing us to dig through large datasets and locate patterns behavior or signals that would be nearly impossible for humans to find. This force multiplier allows technicians at their disposal to identify unusual behaviors. Then the behavioral analysis with UEBA (User Entity Behavior Analytics) tools for safety will do the rest. Mundane and repetitive tasks can also be automated with machine learning, allowing scarce cybersecurity staff resources to focus on higher-value tasks.
UEBA and security
Machine learning and artificial intelligence are based on so-called “big data”. Their efficiency and accuracy improve with the amount of data passed to them . The important thing, however, is to collect the right data. This is where UEBA security systems come in. . The combination of accurate and essential behavioral data with machine learning is the strength of this cybersecurity system. This combination allows you to accurately monitor users, providing deep visibility into what they do on a regular basis .
Once a behavior routine has been established, when a user takes actions that the system considers abnormal , a notification is generated. The operations team is thus notified of any action outside the routines defined . If the detected activity is legitimate, analysts can simply label the activity as part of the routine . Machine learning integrates that data into subsequent data analysis for corporate cybersecurity . The crucial step is normalizing behavior , which ensures that that precise action will no longer generate notifications.
Obviously, in the early stages of system installation, the notifications will be numerous . As AI reduces these false positives by “learning”, whenever moves away from normal behavior, l and notifications become more urgent for safety.
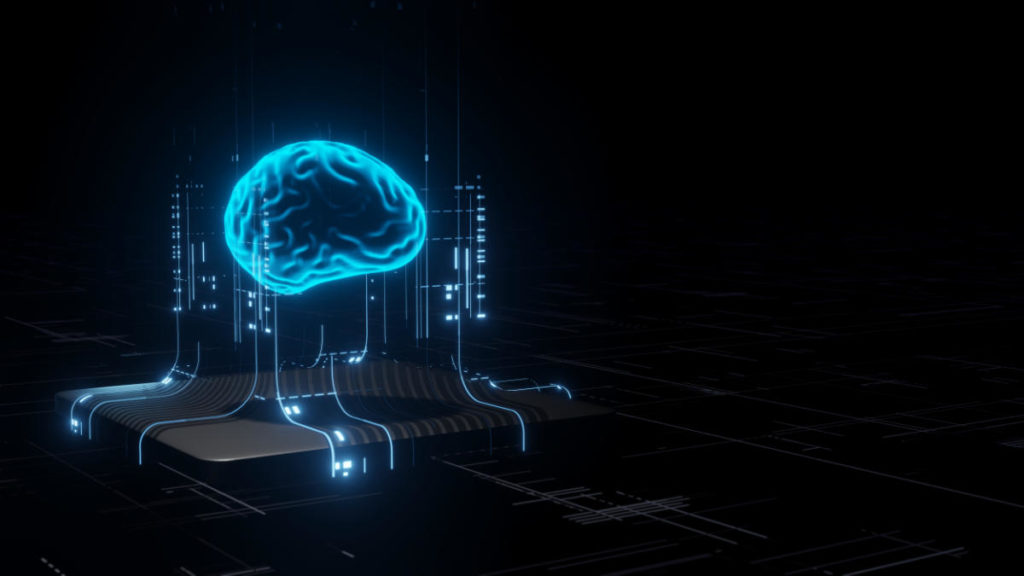
The benefits of combining UEBA, machine learning and security
The use of machine learning together with user behavior data provides a level of proactivity to cybersecurity that is not possible when relying on traditional sign-based detection and prevention systems . This is because you are able to detect subtle changes in behavior that are difficult to detect with traditional systems. It is simply not possible to configure a system with every single permutation of rules to detect all attacks.
Detecting low-level reconnaissance activities using UEBA and machine learning is far more likely to trigger your spider senses than other methods. This provides a huge advantage, making it much more difficult for attackers to evade control by flying under any rules-based radar.
The added value
The advantages of using a UEBA security solution built on a machine learning platform for cybersecurity are many. Because their ability to manage network activity routines is refined, not only they can detect abnormal changes in behavior, but this information can become part of a proactive process , identifying and preventing certain behaviors before they occur.
But perhaps most importantly, machine learning is entering the scene at a very sensitive time for cybersecurity. The number of analysts needed to sift through the data by hand and identify threats is exceeding the number of professionals available. Removing the man from a task for which he is not particularly suitable , is free to focus on areas where it can add value, such as further developing cybersecurity protocols.
So, does machine learning add value to cybersecurity? We really believe so.
Costs and proposed solutions
In terms of costs in general, with a UEBA system maintenance is reduced and does not require the hiring of new professionals, especially if you consider the use of the service in SaaS solution.
We at SOD offer the machine learning and UEBA system, together with a SIEM Next Gen in an ideal solution for those who do not want to invest in the installation of dedicated hardware . Our SOC as a Service is designed to optimize your investment and maximize the return in terms of quality of security procedures.
To find out more, visit the service page or contact us to find out how this solution can be useful for your business. We will answer all your doubts.
Useful links:
Useful links:
UEBA: Behavior Analysis Explained
Customers
Twitter FEED
Recent activity
-
SecureOnlineDesktop
Estimated reading time: 6 minutes L'impatto crescente delle minacce informatiche, su sistemi operativi privati op… https://t.co/FimxTS4o9G
-
SecureOnlineDesktop
Estimated reading time: 6 minutes The growing impact of cyber threats, on private or corporate operating systems… https://t.co/y6G6RYA9n1
-
SecureOnlineDesktop
Tempo di lettura stimato: 6 minuti Today we are talking about the CTI update of our services. Data security is… https://t.co/YAZkn7iFqa
-
SecureOnlineDesktop
Estimated reading time: 6 minutes Il tema della sicurezza delle informazioni è di grande attualità in questo peri… https://t.co/tfve5Kzr09
-
SecureOnlineDesktop
Estimated reading time: 6 minutes The issue of information security is very topical in this historical period ch… https://t.co/TP8gvdRcrF
Newsletter
{subscription_form_1}© 2024 Cyberfero s.r.l. All Rights Reserved. Sede Legale: via Statuto 3 - 42121 Reggio Emilia (RE) – PEC [email protected] Cod. fiscale e P.IVA 03058120357 – R.E.A. 356650 Informativa Privacy - Certificazioni ISO